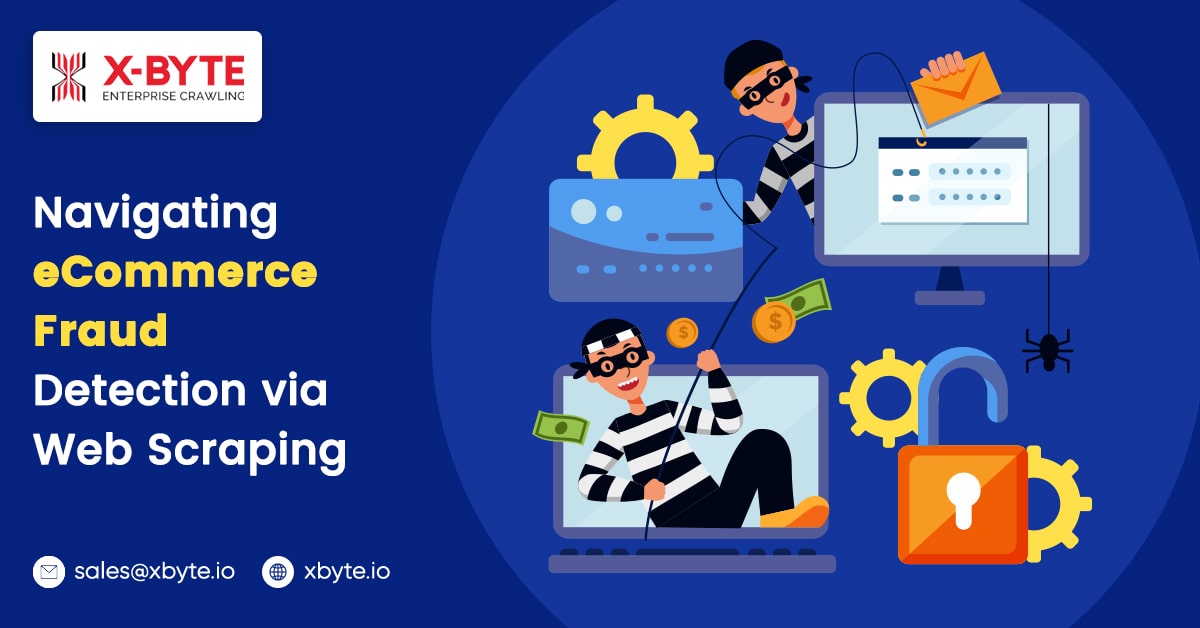
Introduction:
E-Commerce has become an essential part of world retail in the present day. It was projected that retail eCommerce sales would reach more than USD 5.7 trillion worldwide in 2022, and we can only expect those numbers to go through the roof in the near future. There are 9.5 million eCommerce sites in the US alone, so we can only guess how many online retail shops there are in the rest of the world.
But as stores are forced to sell things online and put money into a strong eCommerce presence, they often run into problems with cyber security. Cyber threats make it even harder to stay alive in eCommerce, which is already competitive, fast-paced, and constantly changing. But there are ways that eCommerce retailers can get around the situation. What do they mean? Let us find out!
A Brief About eCommerce Fraud:
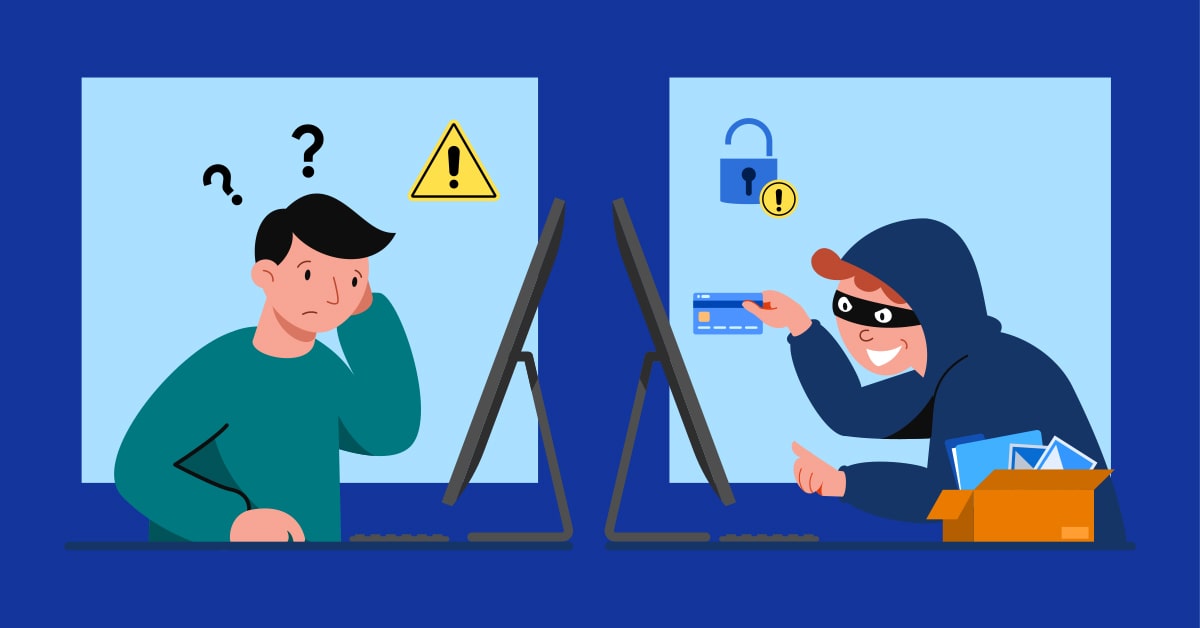
Cyber threats and problems are getting worse as online shops grow. E-commerce fraud is any kind of bad behavior meant to take advantage of online shops. Most attacks try to stop fake transactions made with stolen credit card numbers. Also, eCommerce theft can come in different forms, such as account takeover or return fraud.
In this day and age, every store owner knows that they need to protect themselves from thieves and con artists. During the COVID-19 pandemic, for example, the risk of scams increased by 70%. These shocking numbers are a constant source of worry for eCommerce sellers, whose entire inventory, reputation, and other sources of income can be put at risk by just one malware.
Ways of Detecting Data Fraud in eCommerce:
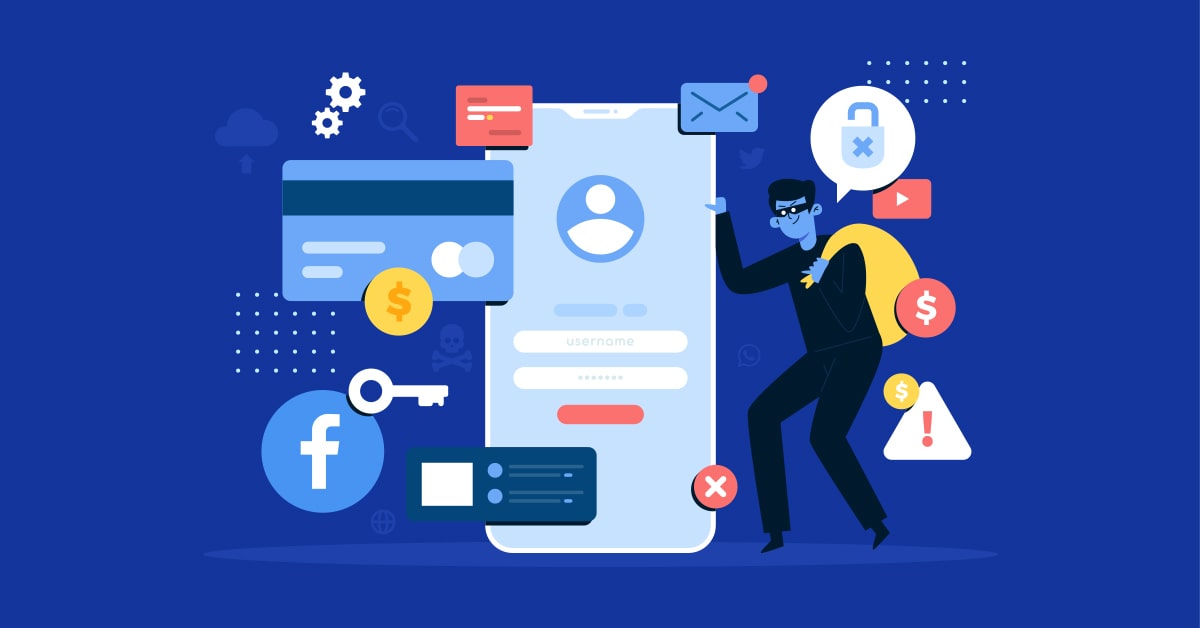
Detecting data fraud in eCommerce is an all-encompassing process that includes tools and methods an online store can use to reduce the costs and resources lost to fraud. It has, among other things, stopped purchases with stolen card numbers and stopped or reduced chargebacks and friendly fraud.
At first, glance, finding eCommerce scams may seem impossible, but there are ways to get around the tricky situation. Fraudsters do everything they can to keep their online actions from being tied to who they are in real life. So, the key is to find out who the customers are before they get to the checkout step, which can help cut down on fraud rates. Some of the things you can do to learn more about your customers and avoid scams are:
- Data Point Comparison: You can find out who a customer is by using the geolocation of their IP and mailing addresses.
- Check for a Social Footprint: If you don’t verify the cardholder’s name, public information from social media can also let shady payments get through.
- Identifying Card Testing Attempts: For most online shops, transaction fraud will show up as small purchases that scammers make to see if the card works. So, keep a close eye on purchases less than USD 1, especially if they come from the same account.
- Understand the Point of Connection: You must know how people connect to your site. This can tell you a lot about how risky it is. For example, customers using VPNs, proxies, and similar tools are more likely to fake data. So, you need to be extra careful when keeping an eye on your eCommerce activities.
How to Build a Fraud Detection Model
Here are the steps you need to take to build a fraud detection tool for eCommerce sites:
1. Establish Project Objectives, Measurement Standards, And Resource Allocation
The first step in making a model to find eCommerce fraud is to figure out what kinds of scams you want to see. Thinking about the types of frauds you wish to find, the critical methods you can use, etc., can help.
2. Identify Proper Data Sources:
After the goals have been approved and shared, you can start to find and collect useful data sources for the fraud detection system. The most common places where info comes from for systems that spot fraud are:
- Client profile
- Risk Profile
- Product Usage
- Billing Data
You can also get more information from third-party data providers. For example, compliance data from the government can be used in the financial business to help build a fraud model.
3. Design the Fraud Detection System Architecture:
When planning the layout of the fraud-detection system, there are a few things to keep in mind, such as–
- Detection Frequency: This is how often the scam scoring model is run on new data
- Fraud-prevention Operation Flow: It affects how and when you mark different events as subscriptions and how you can handle and confirm suspicious ones later.
- Scoring Accuracy: This helps determine if the fraud-scoring model is good enough.
4. Create The Modeling Pipelines, Data Transformation, And Data Engineering:
After the design of the fraud detection model has been made, you can start building the data engineering, transformation, and modeling pipelines.
- For the data engineering pipeline, you need to take data from different sources and combine it. You must also group the data based on business measures and set up batch processes.• For the data engineering pipeline, you need to take data from different sources and combine it. You must also group the data based on business measures and set up batch processes.
- For the second part, the major goal of the data transformation pipelines should be to improve the data quality, deal with problems like missing or wrong data, and change the data so that machine learning models can use it.
- Finally, you can build and compare different machine learning models based on key business measures for the machine learning model pipelines.
5. Integrate the Model into the Case Management System:
Lastly, you need to add the best ML model to the case management system. Using the risk number, you can put each case in order of how risky it is. After that, you’ll get a list of extraordinary circumstances to investigate further.
How to Clean and Preprocess the Scraped Data:
Last but not least, it is vital to have basic knowledge of cleaning and preprocessing scraped data. To achieve the feat, you have to follow the steps given below:
- Remove Irrelevant Data
- Deduplicate Your Data
- Fix Structural Errors
- Deal with Missing Data
- Filter out Data Outliers
- Validate Your Data
Conclusion
So, there you have it: a quick summary of how to use scraped web data to find eCommerce scams. Web scraping is an excellent thing for eCommerce sellers in the modern world, and data can be used to solve many problems, such as fraud and scams.